Table Of Contents
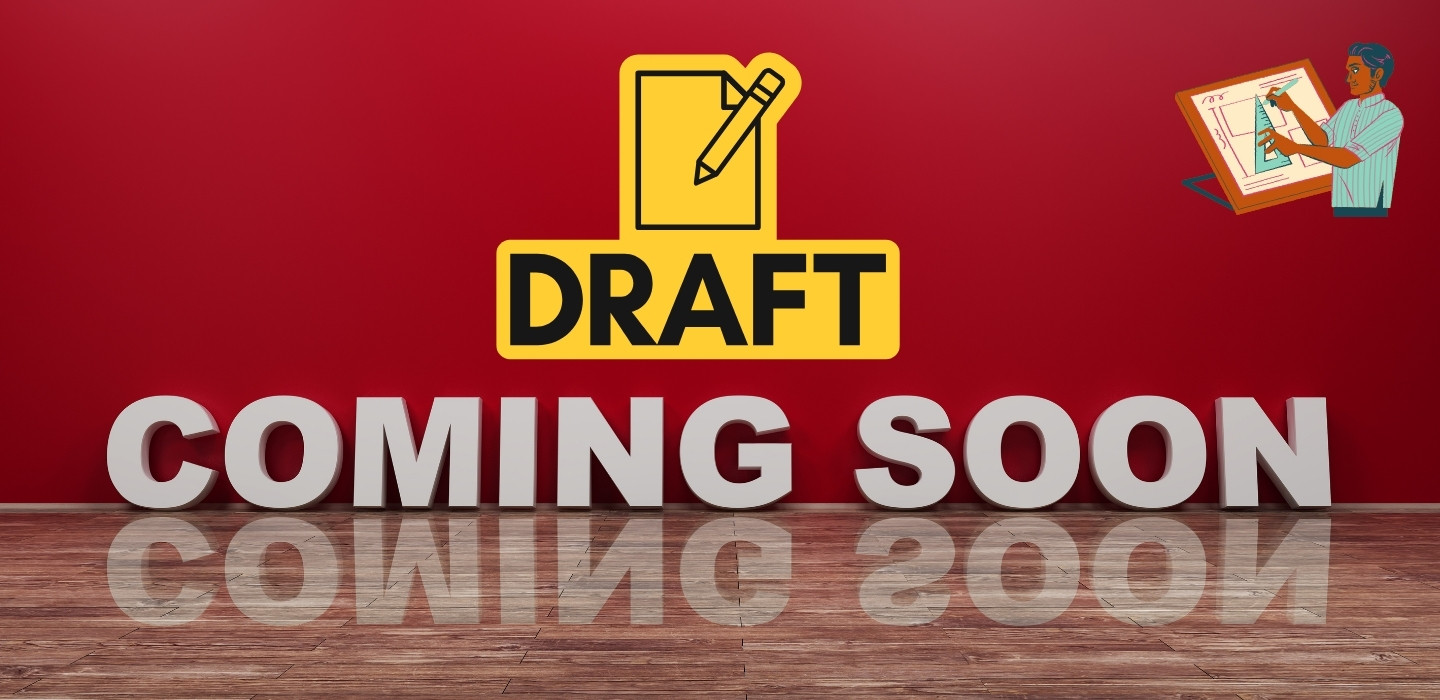
Introduction: Why Tokenization Matters
Artificial Intelligence models process human language by breaking it down into smaller parts. Computers do not understand words the way humans do, so they need a way to convert text into something they can work with. This is where tokenization comes in. It is the foundation of how AI reads, analyzes, and generates text. Without tokenization, models like GPT-4, BERT, and other natural language processing systems would not be able to function effectively.
What is Tokenization?
Tokenization is the process of splitting text into smaller units called tokens. These tokens can be words, characters, or subwords, depending on the approach used. The purpose of tokenization is to create a structured format that AI can process. Once text is broken down into tokens, the model can analyze their relationships, assign meaning, and generate responses based on context.
For example, if you have the sentence “Machine learning is transforming industries,” tokenization might break it down into:
- Word-based tokens: [“Machine”, “learning”, “is”, “transforming”, “industries”]
- Character-based tokens: [“M”, “a”, “c”, “h”, “i”, “n”, “e”, …]
- Subword tokens: [“Machine”, “learn”, “ing”, “is”, “transform”, “ing”, “industries”]
Each method has different use cases and impacts how well AI understands the input.
Types of Tokenization
Word Tokenization
This method splits text into individual words. It is the most straightforward form of tokenization, but it has limitations. Words can have different forms due to variations in grammar, conjugations, and compound words.
For example, the sentence “I am learning tokenization” would be split into [“I”, “am”, “learning”, “tokenization”]. However, this approach struggles with languages that do not use spaces between words, such as Chinese or Japanese.
Subword Tokenization
Subword tokenization addresses the problem of handling rare words and different word forms. Instead of treating each word as a single token, it breaks words into smaller meaningful units. This method helps AI handle words that it has never seen before.
For example, “unhappiness” might be split into [“un”, “happiness”], and “playing” might be split into [“play”, “ing”]. This way, even if “unhappiness” is a new word, the model understands it because it has seen “un” and “happiness” before.
The Byte-Pair Encoding (BPE) algorithm is a popular subword tokenization method used in models like GPT and BERT. It helps strike a balance between efficiency and accuracy.
Character Tokenization
This method breaks text into individual characters. It is useful for languages with complex word structures or when dealing with typos and unknown words. However, character-based models need longer sequences to understand context, which makes them slower and less efficient.
For example, “Tokenization” would be split into [“T”, “o”, “k”, “e”, “n”, “i”, “z”, “a”, “t”, “i”, “o”, “n”].
While this method is useful for certain applications, it is generally less efficient for large-scale AI systems.
Sentence Tokenization
Instead of breaking text into words or subwords, sentence tokenization divides text into full sentences. This is useful for tasks like document summarization, translation, and sentiment analysis.
For example, the paragraph: “Tokenization is important. AI models use it to understand text.” would be split into [“Tokenization is important.”, “AI models use it to understand text.”].
Sentence tokenization is often used before applying word or subword tokenization to structure the input for AI models.
How Tokenization Affects AI Models
Impacts Model Understanding
The choice of tokenization method affects how well an AI model understands language. Subword tokenization allows AI to handle unseen words, making it more flexible. Word tokenization works well for languages with clear word boundaries, while character tokenization is better for handling misspellings and non-standard text.
Affects Memory and Processing Speed
Models process a fixed number of tokens at a time. If a sentence is split into too many small tokens, it increases processing time and memory usage. On the other hand, if tokens are too large, the model might lose important details.
For example, breaking “tokenization” into [“token”, “ization”] instead of [“t”, “o”, “k”, “e”, “n”, …] reduces the number of tokens needed while keeping the meaning intact.
Plays a Key Role in Machine Translation and Chatbots
In translation systems, tokenization helps maintain meaning across languages. A well-tokenized input leads to more accurate translations. In chatbots, tokenization ensures that AI understands and responds in a structured manner.
For example, “What’s the weather like today?” needs to be tokenized correctly so that AI understands the user is asking about the weather and not another topic.
Challenges in Tokenization
Handling Different Languages
Tokenization works well in English because words are usually separated by spaces. However, languages like Chinese, Japanese, and Thai do not have spaces between words, making word tokenization difficult. In these cases, AI relies on language-specific models to segment text properly.
Dealing with Ambiguity
Some words have multiple meanings depending on context. For example, “I saw a bat” could refer to an animal or a baseball bat. Tokenization alone does not solve this, but it prepares text for AI to handle ambiguity later.
Handling Misspellings and Slang
People often write informally, especially on social media. Tokenization needs to handle misspelled words, slang, and abbreviations. For example, “gonna” should be recognized as “going to” in some contexts.
The Future of Tokenization
As AI models continue to evolve, so do tokenization techniques. Newer approaches focus on dynamic tokenization, where models learn the best way to split text based on context. This allows for more efficient processing and better understanding.
Advancements in token-free models are also being explored, where AI can process raw text without predefined tokens. This could improve how AI handles different languages and writing styles.
Final Thoughts: Why Tokenization is the Foundation of AI
Tokenization is the first and most crucial step in how AI processes language. It breaks down text into a format AI can understand, allowing models to generate accurate responses, translate languages, and understand human communication. The choice of tokenization method directly impacts an AI model’s speed, accuracy, and ability to handle complex tasks. As AI technology advances, better tokenization methods will lead to more powerful and efficient models, making AI even more capable in the future.