Table Of Contents
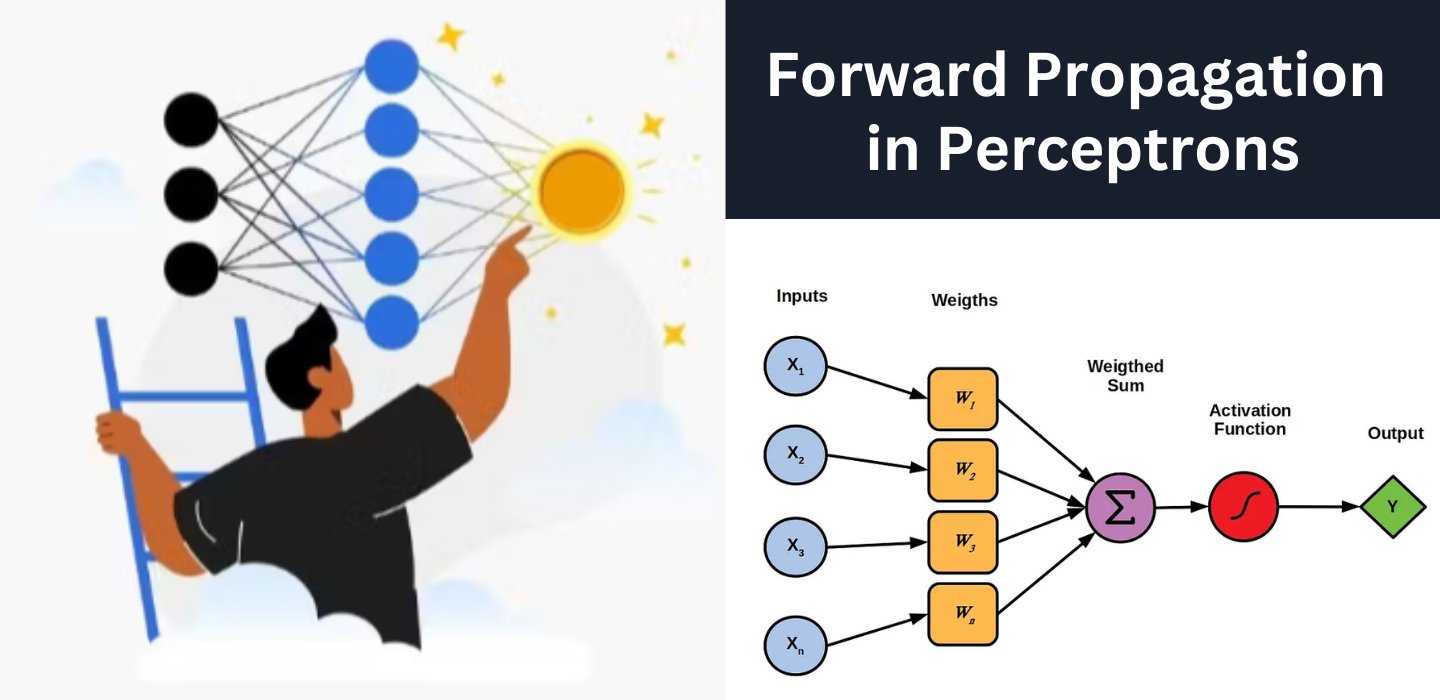
Deep Computer Vision: How AI Sees and Understands the World
Computer vision allows machines to process and interpret images, just like humans. But traditional computer vision relied on manually crafted features, making it limited in complex real-world scenarios. Deep learning changed everything. With deep neural networks, AI can now recognize faces, detect objects, analyze medical scans, and even generate images from text.
How Does Deep Computer Vision Work?
At its core, deep computer vision is powered by convolutional neural networks (CNNs). These networks extract features from images through multiple layers, enabling AI to detect patterns, shapes, and textures. The process follows three key steps:
- Feature Extraction: The AI scans an image, identifying edges, textures, and colors. Early layers focus on simple patterns, while deeper layers detect complex objects.
- Pattern Recognition: Using filters and pooling layers, the model identifies relationships between features like how eyes, nose, and mouth form a face.
- Classification & Decision-Making: The AI assigns labels or makes predictions based on learned patterns, improving accuracy with more training data.
Key Deep Learning Models for Computer Vision
- Convolutional Neural Networks (CNNs): The foundation of deep computer vision. CNNs use convolutional layers to detect spatial patterns in images.
- Recurrent Neural Networks (RNNs) + Vision: Used for image captioning and video analysis, combining visual and sequential data.
- Transformers for Vision (ViTs): A more recent approach where transformers replace CNNs for improved accuracy in image classification.
- Generative Adversarial Networks (GANs): Used for creating realistic images, deepfake generation, and artistic style transfer.
Where Deep Computer Vision is Used Today
Deep learning in computer vision is transforming industries:
- Healthcare: AI detects diseases from medical scans with high accuracy. It identifies tumors, retinal diseases, and even predicts patient outcomes.
- Autonomous Vehicles: Self-driving cars rely on deep vision to detect pedestrians, traffic signals, and road conditions in real time.
- Retail & E-Commerce: AI powers facial recognition for payments, product recommendations based on image searches, and automated checkout systems.
- Manufacturing & Quality Control: AI detects defects in products, ensuring higher quality and reducing manual inspection efforts.
- Security & Surveillance: AI-driven facial recognition and anomaly detection enhance security monitoring worldwide.
Challenges and Limitations of Deep Computer Vision
While deep computer vision has made incredible advancements, it still faces challenges:
- Data Dependency: AI models require vast amounts of labeled data. Poor-quality or biased datasets lead to inaccurate predictions.
- Computational Costs: Training deep networks demands high processing power, requiring GPUs, TPUs, and cloud infrastructure.
- Adversarial Attacks: AI models can be fooled by manipulated images, leading to incorrect classifications or security vulnerabilities.
- Lack of Explainability: Deep learning models work as black boxes, making it difficult to understand why a particular decision was made.
The Future of Deep Computer Vision
With advancements in self-supervised learning, multimodal AI, and real-time vision models, computer vision is set to become even more powerful. AI is moving beyond just classification, it is learning to reason, predict, and interact with visual data in ways that mimic human perception.
Deep computer vision is not just about recognizing objects, it is about understanding the world. The next breakthrough will come when AI can not only see but also comprehend visual context like humans do.
The real question is how far can AI go in bridging the gap between machine perception and human vision?