Table Of Contents
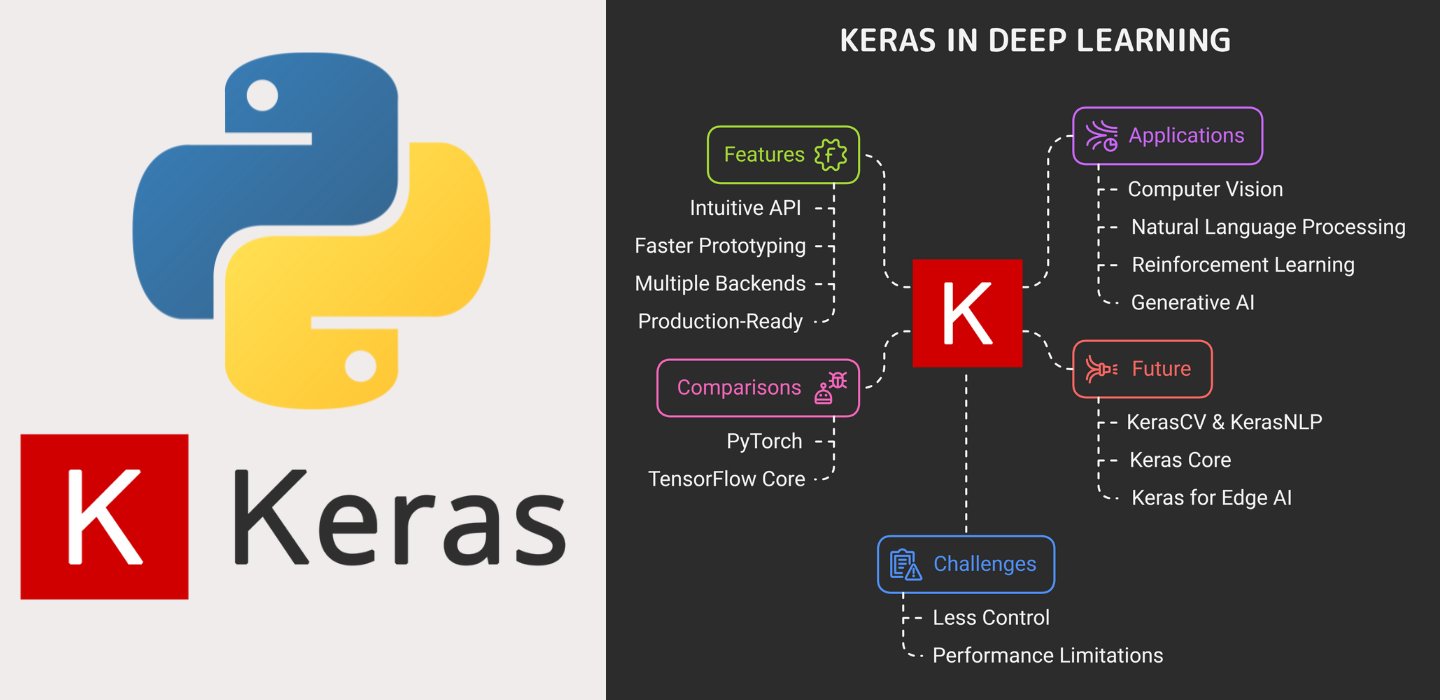
Keras The Hidden Force Behind AI Simplicity and Power
When I first started working with deep learning, everything seemed overwhelming. There were complex neural networks, tensor manipulations, and debugging issues that made the process frustrating. Then, I came across Keras. It changed everything. It made deep learning more accessible and allowed me to focus on solving problems rather than struggling with low-level implementation.
Keras is not just another deep learning framework. It is the bridge between research and real-world applications. It allows developers to create powerful neural networks with minimal code while still offering enough flexibility for advanced AI models.
What Makes Keras Special
To understand the importance of Keras, it is essential to look beyond just the technical details. AI is evolving rapidly, and new frameworks keep emerging. Yet, Keras remains one of the most widely used deep learning libraries.
The reason is simple. It removes unnecessary complexity and makes AI more practical for developers and researchers. It is designed to make deep learning models easier to build, train, and deploy without compromising performance.
The Problem Before Keras
A decade ago, working with deep learning meant dealing with low-level coding and complex computation graphs. If someone wanted to build a neural network, they had to handle everything manually.
This included defining tensor computations, optimizing memory usage, and debugging complex execution errors. This made deep learning less accessible, limiting it to researchers and those with extensive technical expertise.
How Keras Changed Deep Learning
Keras introduced a high-level API that made deep learning accessible to a wider audience. Instead of writing hundreds of lines of code to build a model, developers could now define and train a neural network with just a few lines.
It was not just about reducing the amount of code. It was about making AI development faster, more intuitive, and scalable. The ease of use that Keras brought to deep learning is one of the reasons why it quickly became the preferred choice for developers.
Breaking Down the Core of Keras
Simplicity Without Compromising Depth
Keras provides a simple interface, but that does not mean it lacks power. It allows developers to build models using a sequential API for straightforward architectures and a functional API for more complex designs.
A simple neural network can be created in Keras using just a few lines of code
import tensorflow as tf
from tensorflow import keras
# Define the model
model = keras.Sequential([
keras.layers.Dense(128, activation='relu', input_shape=(784,)),
keras.layers.Dense(10, activation='softmax')
])
# Compile and train the model
model.compile(optimizer='adam', loss='sparse_categorical_crossentropy', metrics=['accuracy'])
model.fit(training_data, training_labels, epochs=10)
This level of simplicity was not possible before Keras.
Flexibility for Innovation
There is a common misconception that Keras is only for simple models. That is not true. While it makes deep learning more accessible, it also supports advanced architectures.
With the functional API and subclassing, Keras enables
- Custom neural network architectures beyond feedforward models
- Multi-input and multi-output models
- Research applications like transformers, generative adversarial networks, and reinforcement learning
It provides the best of both worlds. It is simple enough for beginners yet powerful enough for experienced AI researchers.
Scalability for Real-World AI
AI is not just about building models. It is about deploying them in real-world applications. A deep learning framework is only useful if it can scale efficiently.
Keras integrates seamlessly with TensorFlow, making it possible to deploy AI models across different environments. Models built with Keras can run on
- Cloud platforms using TensorFlow Serving
- Mobile and embedded devices using TensorFlow Lite
- Web applications using TensorFlow.js
This makes Keras a practical choice for both research and production-level AI solutions.
Where Keras is Used Today
Keras is not just for researchers and data scientists. It is used across industries, powering applications in healthcare, autonomous systems, and natural language processing.
Healthcare and Medical AI
Keras is widely used in the healthcare industry for detecting diseases, analyzing medical images, and predicting patient outcomes. It has been instrumental in improving the accuracy and efficiency of diagnostic tools.
Self Driving Cars and Autonomous Systems
Keras is used in self-driving technology to train deep learning models for image recognition, object detection, and decision-making. Autonomous systems rely on AI models built with Keras to process real-time sensor data.
Natural Language Processing
From chatbots to machine translation, Keras powers some of the most advanced natural language processing models. It supports recurrent neural networks and transformers, making it suitable for applications in conversational AI and text generation.
AI Generated Art and Content
Keras is behind many AI-generated art projects. It is used to train generative adversarial networks that create realistic images, music, and even human-like voices.
Challenges and Limitations of Keras
While Keras is a powerful framework, it is not without its limitations.
Less Control Over Low-Level Operations
For developers who need precise control over tensor operations, frameworks like PyTorch may offer better flexibility. Keras abstracts many details, which can be a limitation for certain research applications.
Performance Limitations
For extremely large-scale AI models, lower-level frameworks allow for better performance tuning. Keras prioritizes ease of use, but this sometimes comes at the cost of fine-grained optimization.
Migration to TensorFlow
Since Keras is now integrated into TensorFlow, older versions that supported other backends like Theano are no longer maintained. Developers working with legacy models may need to migrate to TensorFlow’s ecosystem.
The Future of Keras
AI is advancing rapidly, and Keras continues to evolve alongside it.
KerasCV and KerasNLP
New extensions like KerasCV and KerasNLP are making it easier to develop models for computer vision and natural language processing.
Multi Backend Support
Keras Core is introducing support for multiple backends, allowing developers to choose between TensorFlow, JAX, and PyTorch for training models.
Keras for Edge AI
Optimizations for mobile and IoT devices are making Keras models more efficient for edge computing. This is an essential step in bringing AI capabilities to devices with limited processing power.
Final Thoughts
Keras is more than just a deep learning library. It is a shift in how AI is built, tested, and deployed. Whether someone is a researcher, a startup founder, or a software engineer, learning Keras provides a solid foundation in modern AI development.
The real question is how AI will continue to evolve as it scales beyond cloud computing. With Keras at the center of deep learning, what will the next breakthrough in AI development look like?