Table Of Contents
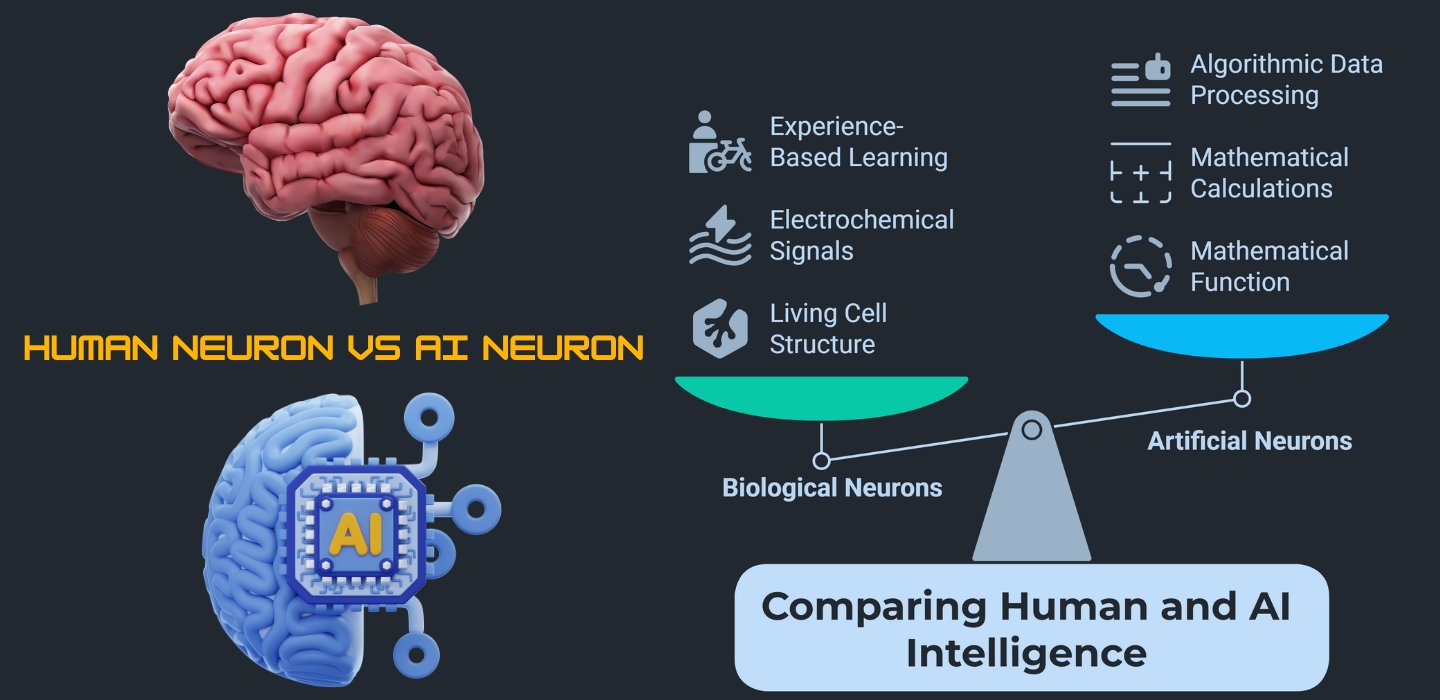
Artificial Neurons vs. Biological Neurons: Understanding the Key Differences
Artificial intelligence is built to mimic human intelligence, but can AI function like the human brain? The foundation of both biological and artificial intelligence comes down to neurons, the basic processing units that handle information. But while artificial neurons try to replicate biological neurons, the two are fundamentally different in structure, function, and adaptability.
What is a Biological Neuron?
A biological neuron is the building block of the human nervous system. The brain contains nearly 86 billion neurons, each forming thousands of connections, enabling us to think, learn, and react in real time.
A biological neuron consists of:
- Dendrites receive electrical signals from other neurons.
- Cell Body (Soma) The processing center where signals are combined and analyzed.
- Axon, A long fiber that transmits signals to other neurons.
- Synapses are the connections where neurotransmitters transfer signals to the next neuron.
Neurons communicate using electrical and chemical signals, adjusting their strength over time. This adaptability is what allows humans to learn, remember, and adapt to new situations.
What is an Artificial Neuron?
An artificial neuron is the basic unit of an artificial neural network (ANN) the technology behind deep learning. It is a mathematical function that processes inputs and produces an output, similar to how biological neurons work.
An artificial neuron follows these steps:
- Receives input data (like images, text, or numerical values) is fed into the neuron.
- Applies Weights Each input has a weight that determines its importance.
- Computes a Weighted Sum The neuron calculates the sum of weighted inputs.
- Applies an Activation Function. This function decides whether the neuron “fires” or remains inactive.
- Generates an Output The processed information is passed to the next layer in the network.
Unlike biological neurons, artificial neurons do not use chemical signals. Instead, they rely purely on mathematical operations and data processing.
Key Differences Between Artificial and Biological Neurons
1. Structure and Complexity
- Biological neurons are highly complex, with billions of dynamic connections that evolve.
- Artificial neurons are simplified mathematical models, designed for efficiency rather than biological accuracy.
2. Speed of Processing
- Artificial neurons operate much faster than biological neurons. A CPU/GPU can process millions of operations per second, while biological neurons communicate at around 200 Hz (or 200 signals per second).
- However, biological neurons are more energy-efficient, consuming just 20 watts to power the entire brain, whereas deep learning models require massive computational power.
3. Learning and Adaptability
- Biological neurons adapt in real-time, changing their structure through synaptic plasticity. This allows humans to learn from a single experience and adjust behaviors instantly.
- Artificial neurons learn through backpropagation, adjusting weights based on errors. However, they require huge amounts of labeled data and thousands of iterations to achieve learning.
4. Memory and Storage
- The brain stores information in a distributed and interconnected way, making it resilient to damage. If some neurons die, others can compensate.
- Artificial neural networks store information in weights and biases, making them vulnerable if a model is corrupted or overfitted, it loses its ability to function correctly.
5. Signal Transmission
- Biological neurons use electrochemical signals, which vary based on hormones, emotions, and external stimuli.
- Artificial neurons use fixed mathematical functions, lacking any adaptation beyond what is programmed.
Which is More Powerful?
Biological neurons far surpass artificial neurons in adaptability, learning efficiency, and contextual awareness. The human brain can generalize, reason, and adapt to new information instantly, while AI struggles with context, common sense, and reasoning beyond data patterns.
However, artificial neurons excel in raw speed and precision. They can process massive datasets in seconds, detect patterns faster than humans, and handle repetitive tasks without fatigue.
The Future of AI: Bridging the Gap
The goal of AI research is not to replace biological intelligence but to combine the strengths of both systems. Advancements in neuromorphic computing and spiking neural networks (SNNs) aim to make artificial neurons more biologically inspired allowing machines to learn faster, adapt better, and use less energy.
As AI evolves, the real question is: Can artificial neurons ever match the adaptability and intelligence of the human brain?