Table Of Contents
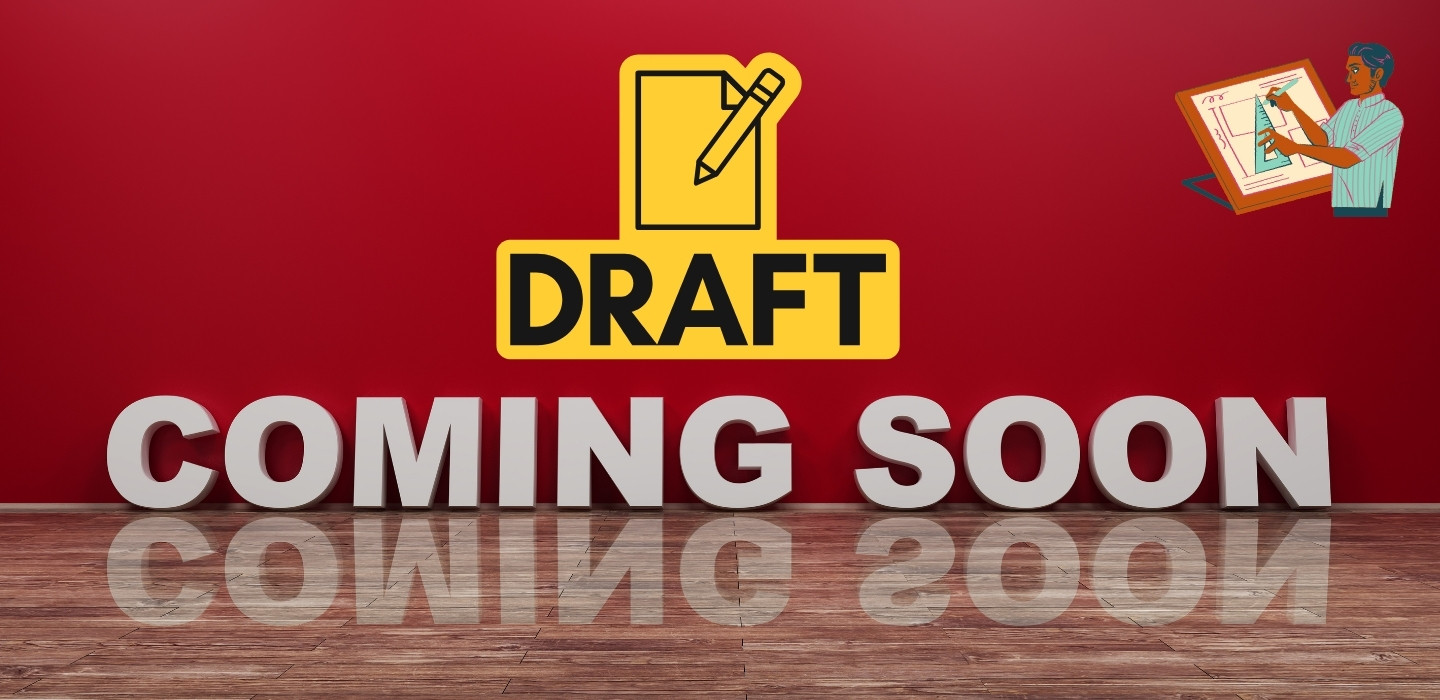
Introduction: What is LLaMA and Why Does It Matter?
Imagine if cutting-edge AI technology was no longer locked behind closed doors but available for researchers, developers, and innovators worldwide. That is exactly what Meta aims to achieve with LLaMA (Large Language Model Meta AI) an open-source, high-performance AI model designed to make artificial intelligence more accessible, scalable, and efficient.
Unlike proprietary models like GPT-4 and Google’s PaLM-2, which require significant computational resources and remain restricted for select users, LLaMA offers a more lightweight and research-friendly alternative. It is optimized to deliver state-of-the-art natural language processing (NLP) performance while keeping hardware requirements low, making AI development more practical and widely available.
How LLaMA Works: The Core Architecture
LLaMA is a transformer-based AI model designed for tasks like text generation, summarization, translation, and reasoning. Like other large language models, it uses deep learning to process and predict text based on input. However, what makes LLaMA different is its focus on efficiency, allowing it to achieve state-of-the-art performance without requiring excessive computational resources.
Pretraining on Diverse Datasets
LLaMA is trained on a large and diverse dataset, including books, scientific papers, and public web data. The goal is to expose the model to a wide range of writing styles, vocabulary, and real-world knowledge. By pretraining on high-quality data, LLaMA improves its ability to understand context, grammar, and sentence structure.
Smaller Model Size, High Performance
Most large AI models require extensive hardware to run, making them expensive and inaccessible to many researchers. LLaMA is optimized to deliver high performance while using fewer parameters compared to models like GPT-4. This makes it more efficient, reducing the need for massive data centers while still providing strong language capabilities.
Transformer-Based Architecture
Like GPT-based models, LLaMA uses the transformer architecture, which processes text efficiently using self-attention mechanisms. These mechanisms allow the model to analyze relationships between words across long sentences, ensuring that responses are coherent and contextually accurate.
Open-Source Access for Research and Development
One of LLaMA’s key advantages is that it is available to researchers and developers. Unlike proprietary AI models that are locked behind APIs or paid subscriptions, LLaMA allows the AI community to experiment, fine-tune, and improve the model for specific use cases. This has led to rapid innovation, with many researchers building applications and improving its capabilities.
Why LLaMA is a Game-Changer in AI Research
Accessibility for AI Development
Proprietary AI models are expensive to train and run, limiting access to well-funded companies. LLaMA breaks this barrier by providing a powerful yet lightweight model that researchers, universities, and startups can use without heavy infrastructure costs.
Strong Performance with Fewer Resources
LLaMA proves that bigger is not always better in AI. It delivers competitive results while being computationally efficient, allowing it to be deployed on smaller machines without requiring large-scale GPUs.
Faster Adaptation for Different Use Cases
Since LLaMA is open-source, developers can fine-tune it for specific industries such as healthcare, finance, education, and legal analysis. This means businesses can create custom AI applications that match their needs without relying on closed AI models.
Encouraging Transparency in AI Development
Many AI models operate as black boxes, with little insight into how they function. LLaMA promotes transparency by allowing researchers to study its architecture, improving fairness, ethics, and bias reduction in AI.
How LLaMA Compares to Other AI Models
LLaMA vs GPT-4 (OpenAI)
- Efficiency: LLaMA is optimized for lower resource usage, while GPT-4 requires high-end infrastructure.
- Access: LLaMA is open-source, while GPT-4 is proprietary and accessible only via paid APIs.
- Customization: LLaMA allows researchers to fine-tune it, while GPT-4 is controlled by OpenAI.
- Performance: GPT-4 has a larger dataset and model size, giving it a broader knowledge base, but LLaMA remains competitive in many NLP tasks.
LLaMA vs PaLM-2 (Google)
- Scalability: Google’s PaLM-2 is built for large-scale AI applications, while LLaMA is optimized for efficiency and accessibility.
- Research Use: LLaMA is designed for academic and research purposes, while PaLM-2 is integrated into Google’s AI services.
- Open-Source Advantage: LLaMA allows customization and adaptation, whereas PaLM-2 is closed-source.
Real-World Applications of LLaMA
AI Chatbots and Virtual Assistants
LLaMA can power chatbots that understand natural language, provide recommendations, and assist users in various industries like customer service and healthcare.
Educational Tools and AI Tutors
Since it is lightweight and efficient, LLaMA can be deployed in education platforms to provide real-time tutoring, essay feedback, and language translation assistance.
Medical Research and AI-Driven Diagnosis
By fine-tuning LLaMA on medical datasets, researchers can use it for disease prediction, medical summaries, and healthcare automation.
Legal Document Analysis and Contract Review
Legal professionals can use LLaMA-powered tools to analyze contracts, summarize legal texts, and provide insights on case laws, reducing time spent on manual research.
Code Generation and Software Development
LLaMA can assist developers by generating code snippets, debugging programs, and explaining complex algorithms, making software development more efficient.
Challenges of LLaMA and Areas for Improvement
Handling Bias in AI Models
Since LLaMA learns from public datasets, it may inherit biases present in the data. Researchers must implement bias reduction techniques to ensure fair and ethical AI outputs.
Need for Continuous Improvement
AI evolves quickly, and while LLaMA is efficient, newer models with better optimizations will continue to emerge. Researchers need to keep improving LLaMA’s performance and capabilities.
Fine-Tuning for Domain-Specific Applications
While LLaMA is powerful, it may require additional training and fine-tuning to perform well in specialized fields such as law, healthcare, and finance.
The Future of LLaMA and Open-Source AI
LLaMA represents a major shift in AI development by prioritizing accessibility, efficiency, and research collaboration. As AI continues to expand, models like LLaMA will drive innovation in industries that require smart, cost-effective AI solutions.
Future advancements may include:
- LLaMA-powered multimodal AI that can process text, images, and speech.
- Lighter, mobile-friendly versions optimized for edge computing.
- Better fine-tuning tools to help businesses create specialized AI applications.
LLaMA is not just another AI model it is a step towards making powerful AI accessible to a global community of researchers, developers, and businesses.
Final Thoughts: Why LLaMA is a Game-Changer
LLaMA is redefining AI accessibility by providing an open, efficient, and research-friendly model that competes with larger, proprietary systems. Its ability to deliver high performance with lower resource demands makes it a valuable tool for businesses, researchers, and developers looking to build advanced AI applications.
As AI adoption grows, LLaMA will continue shaping the future of NLP and deep learning, proving that cutting-edge AI does not have to be locked behind closed doors.