Table Of Contents
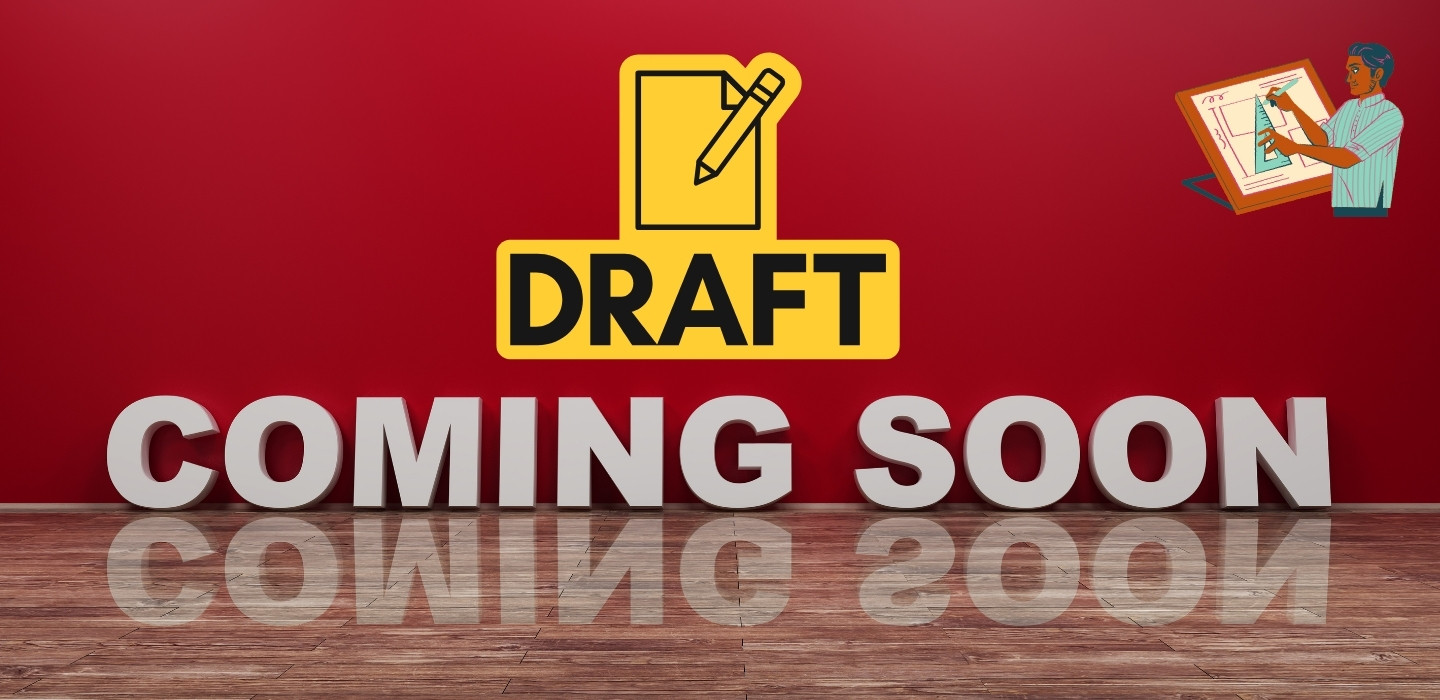
LSTM: Solving AI’s Memory Problem and Transforming Deep Learning
Artificial intelligence has always struggled with memory. Early neural networks could process data step by step, but they had a major weakness they forgot important details too quickly. This made them unreliable for tasks like speech recognition, language translation, and predicting future trends. Then came Long Short-Term Memory (LSTM), a breakthrough in deep learning that gave AI the ability to remember and learn from past data over long sequences.
LSTM is a type of Recurrent Neural Network (RNN), but unlike traditional RNNs, it is specifically designed to solve the short-term memory problem. Standard RNNs could only remember information for a few steps before older details faded. This made them ineffective for understanding long sentences, following complex conversations, or analyzing patterns in lengthy data sequences. LSTM changed that by introducing a system that decides what to remember, update, or discard just like how the human brain filters useful and unnecessary information.
Why Traditional RNNs Were Not Enough
Before LSTMs, AI models relied on RNNs to process sequences of data. While they worked for short-term tasks, they faced a serious problem known as the vanishing gradient. As these models processed more steps, earlier information gradually faded away, making them unreliable for tasks that required long-term understanding.
For example, in a sentence like “The doctor who saved thousands of lives won an award last night,” a traditional RNN might forget that “doctor” is the subject by the time it reaches “won an award.” This loss of information made it difficult for AI to understand complex relationships in text, speech, and time-series data. LSTM solved this problem by allowing AI to retain important details over long sequences, making it far more reliable.
How LSTM Works
LSTM introduces a memory cell and three specialized gates that control how information flows through the network. Instead of automatically overwriting memory at every step (like RNNs do), LSTMs carefully decide what to keep, update, or forget.
The Cell State: AI’s Long-Term Memory
The cell state acts like a conveyor belt, carrying important information across time steps without unnecessary changes. This allows LSTMs to store long-term dependencies, just like how people remember important details while reading a book or following a conversation.
The Forget Gate: Filtering Out Unnecessary Information
Not all information is useful forever. The forget gate decides what details to remove from memory based on relevance. It assigns a value between 0 and 1 to each piece of data. A value closer to 0 means the information is discarded, while a value closer to 1 means it is retained. This ensures that only important details stay in memory, while irrelevant ones are removed.
The Input Gate: Storing New Information
When new data comes in, the input gate evaluates it and decides what should be added to memory. This prevents the AI from being overloaded with unnecessary details while ensuring it learns useful information from ongoing inputs.
The Output Gate: Producing Meaningful Results
Finally, the output gate determines what information should be used to generate the AI’s response. This ensures that the model only outputs relevant, context-aware information instead of random or incomplete details.
Together, these mechanisms allow LSTMs to remember key information over long sequences while continuously learning and adapting to new inputs something traditional RNNs could never do effectively.
Why LSTMs Matter
They Solve the Long-Term Dependency Problem
LSTMs allow AI to retain context over extended sequences, making them essential for applications like language modeling, time-series forecasting, and real-time decision-making.
They Handle Complex Sequential Data
LSTMs are widely used in areas that require AI to understand time-based relationships, such as speech recognition, translation, and predictive analytics.
They Improve Over Time
Unlike traditional models that forget past mistakes, LSTMs can learn from experience, making them a key part of recommendation systems, self-driving cars, and advanced AI assistants.
Real-World Applications of LSTMs
Speech Recognition and Virtual Assistants
LSTMs power voice-based AI systems like Siri, Google Assistant, and Alexa, helping them understand natural conversations, follow context, and respond intelligently. Without LSTMs, voice assistants would struggle with long queries or lose track of previous interactions.
Language Translation
LSTMs improve tools like Google Translate by capturing the meaning of entire sentences rather than translating words individually. This results in more natural and fluent translations.
Predictive Text and Autocorrect
When you type on your phone, predictive text and autocorrect features rely on LSTMs to suggest the next word based on your writing habits. These models learn patterns over time, making predictions more accurate and personalized.
Financial Forecasting and Stock Market Analysis
LSTMs are widely used in finance and trading to detect trends, analyze time-series data, and predict stock prices. They help traders make better decisions by identifying patterns in financial markets.
Healthcare and Medical Diagnosis
AI models powered by LSTMs assist in medical diagnosis and patient monitoring by analyzing health records and predicting potential diseases based on past data. This improves early detection and helps doctors provide more accurate treatments.
Challenges and Limitations of LSTM
Computational Complexity
LSTMs require more computing power than traditional RNNs due to their advanced memory mechanisms. Training these models can be slow, making them challenging for real-time applications.
Struggles with Very Long Sequences
While LSTMs are much better than RNNs, they still face difficulties when processing extremely long sequences. As data grows, LSTMs require more memory, which can slow down performance.
Replaced by Transformers in Many Areas
LSTMs were once the best solution for handling sequences, but newer models like Transformers (used in GPT-4, BERT, and Google’s PaLM-2) have outperformed LSTMs in many tasks. Transformers use self-attention, which allows them to process entire sequences in parallel rather than step by step. However, LSTMs are still valuable for real-time processing, lower-cost AI applications, and tasks requiring fewer resources.
The Future of LSTMs in AI
Even though Transformers have taken over many areas, LSTMs are still widely used for real-time AI systems, speech recognition, and predictive analytics. Researchers are also developing hybrid models that combine LSTMs with newer architectures, offering the best of both worlds.
Memory-based learning remains essential for AI, whether it is chatbots remembering past interactions, predictive text adapting to user behavior, or AI-driven medical tools analyzing patient history. LSTMs continue to be an important building block in deep learning, even as AI evolves into more complex architectures.
Final Thoughts: Why LSTMs Still Matter
LSTMs revolutionized AI by giving machines the ability to remember, learn, and improve over time. They solved the short-term memory problem of RNNs and became the foundation for smarter AI applications in speech recognition, translation, finance, and healthcare.
While newer models like Transformers have taken over some areas, LSTMs remain a critical tool for sequential data analysis and real-time AI. AI will keep evolving, but the fundamental idea behind LSTMs teaching machines how to remember and learn from experience will always be at the heart of intelligent systems.