Table Of Contents
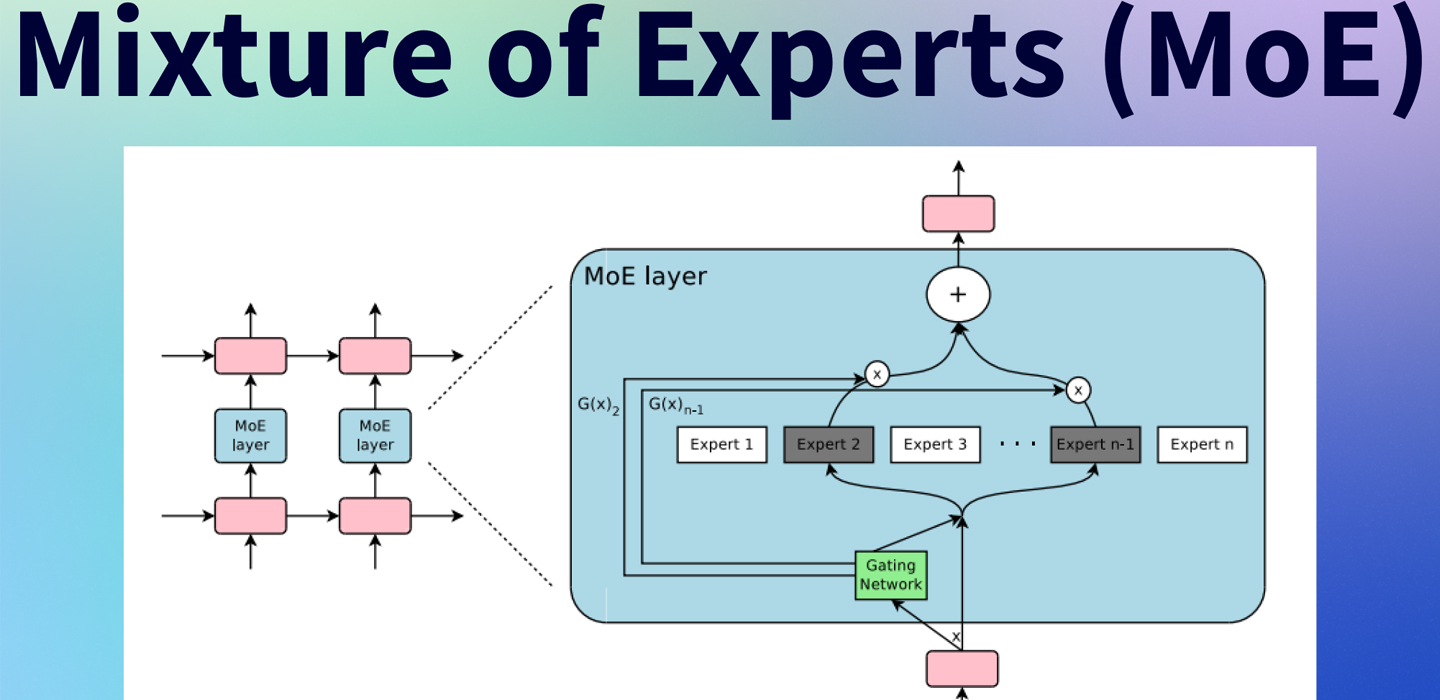
Image credit: Hugging Face
Introduction: Making AI More Efficient
As artificial intelligence (AI) models get bigger and more advanced, they also require more computing power, memory, and energy. Large models like GPT-4 and Google’s PaLM-2 process every request using their entire network, which leads to high costs, slower responses, and more energy consumption.
Instead of using all the model’s resources for every task, Mixture-of-Experts (MoE) provides a more efficient and intelligent approach. It allows AI models to activate only the parts needed for a specific task, making them faster, smarter, and less expensive to run.
MoE is more than just an upgrade it changes how AI models are built and scaled, improving accuracy while keeping efficiency in check.
How Does Mixture-of-Experts Work?
MoE divides a large AI model into smaller, specialized sections called “experts.” Each expert focuses on specific types of tasks, and instead of using the entire model for every request, MoE chooses only the experts needed for that task.
The Three-Step Process of MoE:
- Processing the Input
- When an AI receives a request, it analyzes the question or task.
- Instead of using the whole model, a component called the router decides which experts are most suited for the request.
- Selecting and Activating Experts
- The router picks a small group of experts (usually two to four) from a larger pool of trained experts.
- Each expert works on a different part of the problem based on its specialization.
- Generating the Output
- The chosen experts process the input separately and generate responses.
- Their outputs are combined into a final, clear answer before being sent back to the user.
This method allows AI models to be highly efficient, using only the necessary computing power instead of engaging the full model for every task.
Why Mixture-of-Experts is a Game-Changer for AI
Better Scaling Without Increasing Costs
AI models are growing in size, but scaling them up usually makes them more expensive to run. MoE avoids this problem by activating only the needed experts, meaning AI can handle complex tasks without draining resources.
For example, Google’s Switch Transformer, which uses MoE, is as accurate as traditional AI models but requires seven times less computing power per request.
More Accurate and Specialized AI Responses
Instead of making one AI model try to know everything, MoE allows different experts to specialize in different areas. This makes AI better at answering complex or technical questions.
For example, a medical AI using MoE could:
- Send cancer-related questions to an expert trained in oncology.
- Route prescription-related questions to an expert in pharmacology.
- Direct radiology questions to an expert specializing in image analysis.
By assigning tasks to the right experts, MoE ensures higher accuracy and reliability.
Faster and More Energy Efficient
AI models consume large amounts of electricity, which increases costs and affects the environment. MoE helps by using only a fraction of the model at a time, reducing power consumption while maintaining high performance.
This is important for companies that want AI-powered applications without huge operating costs.
Where is Mixture-of-Experts Being Used?
AI Chatbots and Virtual Assistants
AI assistants like chatbots need to answer many types of questions. MoE can route different questions to the right expert:
- Finance-related queries go to an expert trained in financial services.
- Legal questions are handled by an expert trained in law and regulations.
- Product-related support queries are answered by an expert in customer service.
This reduces errors and makes interactions feel more natural.
AI-Powered Code Generation
AI-powered coding assistants like GitHub Copilot or DeepMind AlphaCode can use MoE to assign coding tasks to experts specializing in different programming languages.
Instead of treating every programming language the same way, MoE could:
- Send Python-related problems to a Python expert.
- Assign JavaScript-related tasks to an expert in front-end development.
- Direct C++ optimization tasks to an expert trained in performance tuning.
This results in more optimized and accurate code suggestions.
Medical Diagnosis and Healthcare AI
Medical AI must be highly accurate, and MoE can help by directing different types of medical questions to the right experts.
For example, an MoE-based healthcare AI could:
- Assign cancer diagnosis cases to an expert trained in oncology.
- Direct drug-related queries to an expert in pharmaceuticals.
- Process genetic research questions through an expert in molecular biology.
This ensures AI-generated medical advice is accurate, up-to-date, and specialized.
Fraud Detection and Financial Forecasting
Banks and financial institutions can use MoE-powered AI to:
- Assign fraud detection tasks to experts trained in identifying suspicious transactions.
- Send investment queries to an expert focused on stock market trends.
- Route risk assessment questions to an expert specializing in financial analysis.
This improves financial security and decision-making.
Challenges of Mixture-of-Experts
Despite its advantages, MoE comes with a few technical challenges:
Training and Optimization is Complex
- The router must be well-trained to select the correct experts for each task.
- If the router picks the wrong experts, results can be inaccurate.
Some Experts May Be Overused or Underused
- Some experts handle too many requests, while others are rarely used.
- Ensuring that all experts are trained and balanced properly is a challenge.
Potential Slowdowns if Experts Are Not Managed Well
- If too many requests go to the same expert, it can create a bottleneck and slow down responses.
- Advanced load balancing strategies are required to keep MoE models running smoothly.
Even with these challenges, MoE research is advancing quickly, and newer models are becoming more efficient and easier to manage.
What’s Next for MoE?
As AI continues to evolve, MoE will play a major role in shaping the future of AI development. Here’s what we can expect in the coming years:
- Self-Learning MoE Models – Future AI will learn which experts to use dynamically, improving efficiency over time.
- Multimodal MoE – AI models will process text, images, and voice together, routing different types of input to specialized experts.
- Decentralized MoE AI – AI models could be spread across multiple devices, reducing dependency on massive data centers.
As AI adoption grows, MoE will help companies build smarter AI that can handle more tasks while keeping costs low.
Final Thoughts: Why MoE is the Future of AI
Mixture-of-Experts (MoE) is changing the way AI models process information, making them faster, smarter, and more efficient. By activating only the required experts, MoE reduces computing costs, improves accuracy, and makes AI more scalable.
With applications in healthcare, finance, customer service, and software development, MoE is reshaping AI into a more practical and specialized tool.
As AI continues to evolve, MoE will be one of the most important breakthroughs in AI architecture, helping create powerful, cost-effective, and highly specialized AI systems for the future.