Table Of Contents
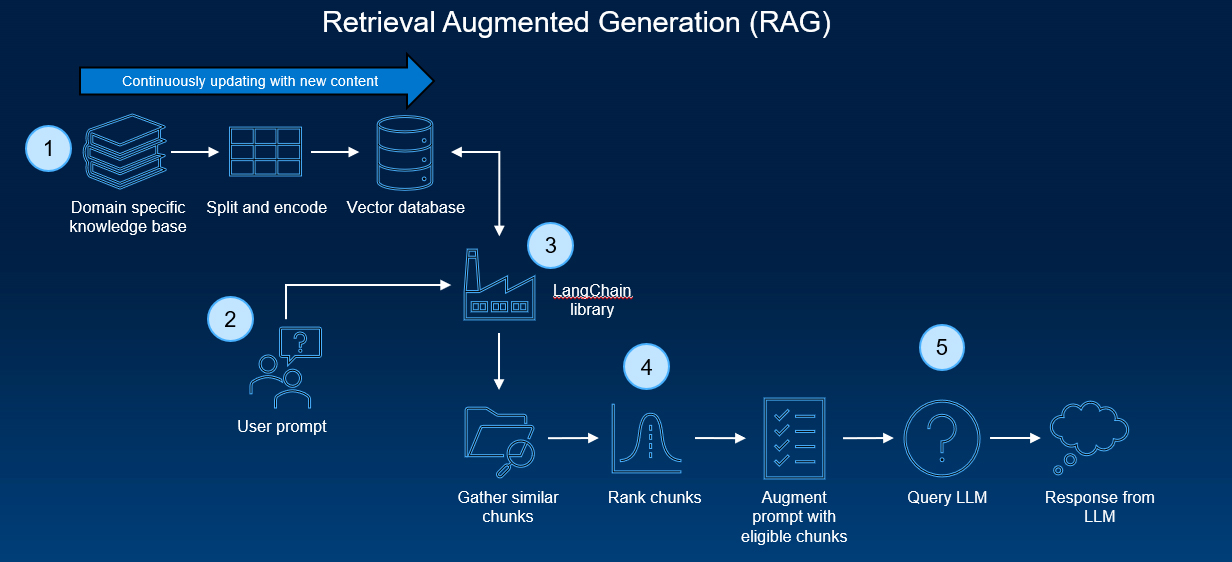
Image credit: Dell Technologies
Introduction: The Need for Smarter AI Responses
Artificial Intelligence has transformed the way we interact with information. Traditional AI models rely on pre-trained knowledge, meaning they generate responses based only on what they’ve learned during training. However, this approach has limitations it can lead to outdated or incorrect answers, especially when dealing with real-time data, domain-specific queries, or complex reasoning tasks.
This is where Retrieval-Augmented Generation (RAG) comes in. RAG enhances Large Language Models (LLMs) by allowing them to fetch relevant, up-to-date information from external sources before generating responses. This significantly improves accuracy, relevance, and factual consistency, making AI much more reliable for real-world applications.
What is Retrieval-Augmented Generation (RAG)?
RAG is a hybrid AI approach that combines two key processes:
- Retrieval: The AI model first searches for relevant information from an external database, knowledge base, or web source.
- Generation: The retrieved data is then used to generate a more accurate, fact-based response.
Unlike traditional AI models that only rely on pre-trained knowledge, RAG dynamically fetches data before responding, ensuring it can adapt to new information and provide contextually relevant answers.
Example:
Imagine asking an AI assistant, “What are the latest advancements in quantum computing?”
- A standard AI model might provide outdated information if it was last trained months ago.
- A RAG-based AI would first retrieve the latest research papers, news articles, or relevant documents before generating a response that reflects the most recent developments.
How Does RAG Work?
The RAG process can be broken down into three key stages:
1. Query Processing & Information Retrieval
When a user asks a question, the AI first identifies keywords and context to understand what kind of information is needed. It then performs a search across external knowledge sources like:
- Internal Databases: Private company knowledge bases, customer support documents.
- Public Knowledge Repositories: Wikipedia, ArXiv, research papers.
- Real-Time Web Search: Live news sources, stock market updates, or financial reports.
2. Relevant Data Selection
Once multiple sources are retrieved, the AI ranks the results based on relevance, credibility, and context-matching. This prevents unreliable sources from influencing responses.
3. AI-Powered Response Generation
After selecting the most relevant content, the AI generates a well-structured, human-like answer.
- It ensures coherence and fluency by integrating retrieved information smoothly into the response.
- If needed, it summarizes long documents into concise, easy-to-understand explanations.
Why is RAG Important?
1. Improves Accuracy and Reduces Hallucinations
One major challenge with LLMs is that they sometimes generate hallucinations plausible but incorrect responses. By fetching verifiable data before generating an answer, RAG dramatically reduces misinformation and increases factual correctness.
2. Enables Domain-Specific AI Assistants
- In healthcare, RAG-based AI can retrieve the latest medical research and treatment guidelines.
- In finance, it can provide real-time stock analysis instead of relying on outdated data.
- In legal tech, it can reference laws, regulations, or case precedents to ensure compliance.
3. Supports Personalized AI Experiences
RAG can be integrated with personal knowledge bases to provide custom responses based on company policies, customer interactions, or internal workflows a game-changer for enterprises.
4. Scalable for Real-World Applications
- Chatbots and virtual assistants become more informative and reliable.
- Enterprise AI systems can access proprietary documents securely while generating responses.
- AI tools for research, education, and journalism can dynamically source the latest reports.
Challenges and Considerations
While RAG improves AI responses significantly, it also presents challenges:
1. Data Source Reliability
The quality of generated responses depends on the reliability of retrieved sources. If the AI fetches information from biased or incorrect sources, it can still produce misleading answers. Ensuring high-quality, verified sources is critical.
2. Latency and Speed
Fetching real-time data adds processing time, which can slow down response generation. Optimizing retrieval without compromising speed is an ongoing challenge in RAG-based AI systems.
3. Security and Privacy Concerns
When RAG accesses external data sources, security must be tightly controlled to prevent leakage of sensitive or proprietary information. Data governance policies and encrypted retrieval systems are crucial for enterprise adoption.
Future of RAG in AI Development
RAG is still evolving, but its impact is already visible in next-generation AI applications.
Upcoming Advancements in RAG
- Better Context-Aware Retrieval: AI will improve at selecting only the most relevant sections of documents instead of retrieving unnecessary information.
- Multimodal RAG: Future models will not only retrieve text but also images, videos, and audio to provide richer responses.
- Self-Learning Retrieval Systems: AI will learn which sources are most reliable over time and prioritize them automatically.
In Summary: A Smarter, More Reliable AI
Retrieval-Augmented Generation (RAG) is a game-changer in AI, allowing models to fetch real-time, fact-based data instead of relying solely on pre-trained knowledge. It enhances accuracy, reduces misinformation, and enables highly personalized AI applications across industries.
As AI adoption continues to grow, RAG-powered models will set new standards for intelligent, context-aware, and trustworthy AI responses. Businesses, researchers, and developers who leverage RAG will be at the forefront of the AI revolution.
The future of AI isn’t just about knowing it’s about retrieving the right knowledge at the right time and generating accurate insights that matter.