Table Of Contents
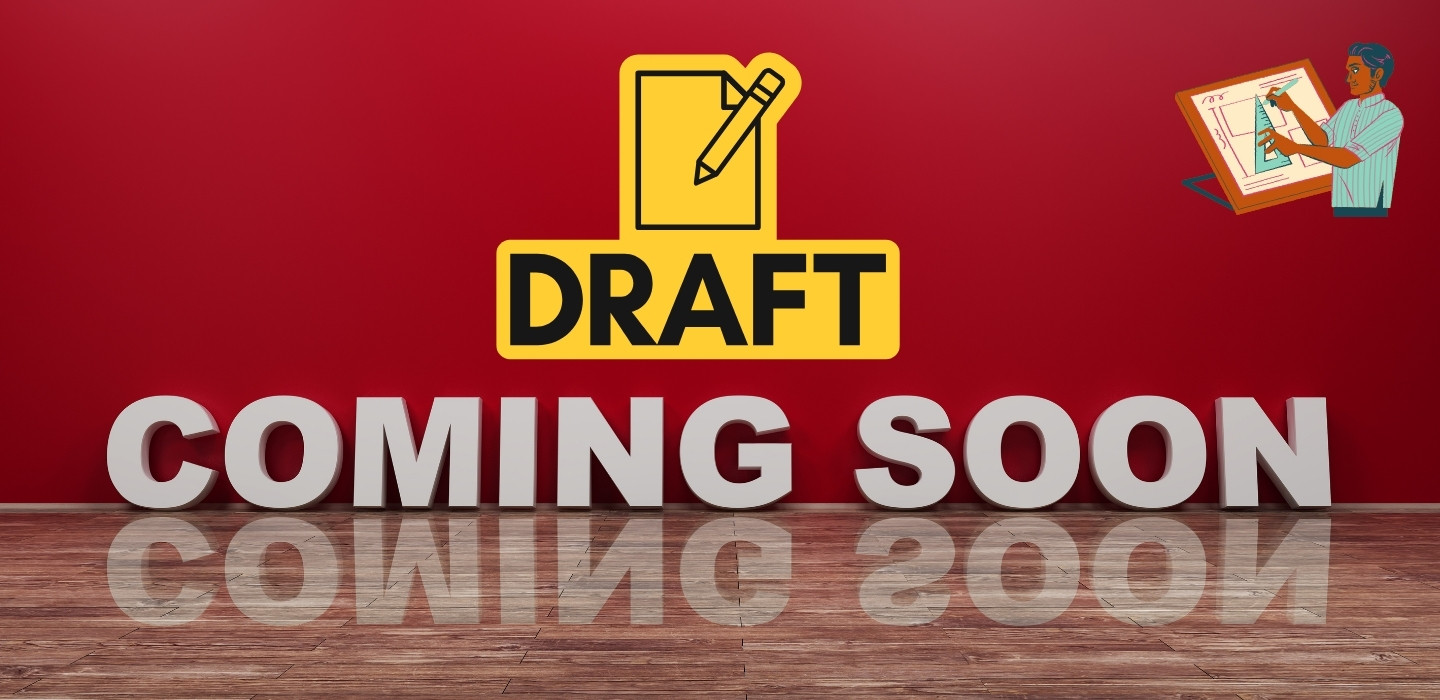
Image credit: Dell Technologies
Introduction: The Shift from Information Retrieval to Intelligent Reasoning
Artificial intelligence is rapidly evolving. While Large Language Models (LLMs) like GPT-4, Claude, and others have shown remarkable progress in understanding and generating human-like responses, they still face limitations in reasoning and fact-based accuracy.
Traditional AI models are only as good as their training data. They generate responses based on patterns and probabilities, often leading to misinformation when the model lacks the right context or knowledge. This is where Retrieval-Augmented Generation (RAG) came in allowing AI to fetch external information before generating responses.
But even RAG has limitations. It improves factual accuracy but does not enhance reasoning or multi-step problem-solving. Enter Retrieval-Augmented Reasoning (RAR) an advanced AI approach that not only retrieves relevant knowledge but also applies structured, multi-step reasoning to arrive at well-thought-out responses.
RAR is the next big leap in AI, enabling models to retrieve, analyze, and reason through complex problems just like a human expert would.
What is Retrieval-Augmented Reasoning (RAR)?
Retrieval-Augmented Reasoning (RAR) is an AI framework that enhances both factual retrieval and logical reasoning. It combines two critical processes:
- Retrieval: The AI first searches for relevant, external information from databases, APIs, research papers, or the web.
- Reasoning: Instead of simply summarizing or regurgitating data, the AI analyzes and processes the retrieved information using logical and structured reasoning techniques.
This means RAR-based AI can handle complex, multi-step reasoning tasks that traditional AI struggles with.
For example:
A standard AI query like “Who won the Nobel Prize in Physics in 2024?” may result in incorrect responses if the training data is outdated. A RAG model will retrieve the latest Nobel Prize winners from a trusted source, but a RAR model will not only retrieve the winner’s name but also analyze their contribution, compare it with past winners, and explain why their work is significant.
A more complex query like “Compare the inflation trends in the US and Europe over the last five years and predict how they will change in the next two years.” would challenge traditional AI due to a lack of updated data. A RAG model will retrieve inflation data but may not analyze trends effectively, while a RAR model will fetch the latest data, compare historical patterns, apply reasoning based on economic indicators, and offer a logical prediction with supporting arguments.
How Does RAR Work?
RAR follows a structured three step process:
1. Query Analysis & Context Understanding
The AI first understands the intent of the query. It breaks down complex multi-step questions into smaller, logical components and determines what kind of information is needed, such as financial reports, scientific research, or real-time data.
2. Intelligent Retrieval of Information
Instead of relying solely on pre-trained knowledge, RAR fetches real-time, relevant data from trusted sources such as research papers (e.g., ArXiv, PubMed), news databases (e.g., Reuters, Bloomberg), government and legal documents, and API-based real-time financial or industry-specific reports. The retrieved data is then filtered and ranked based on credibility, relevance, and context.
3. Structured Reasoning and Response Generation
RAR does not just summarize the retrieved data it processes, evaluates, and applies reasoning to extract meaningful insights. The AI then connects the dots between different data points, synthesizing information in a logical, structured, and easy-to-understand format. The final response resembles expert-level analysis, offering more than just factual correctness it delivers informed reasoning and context-aware predictions.
Why is RAR a Game-Changer for AI?
Moves Beyond Just Retrieval It Thinks Like an Analyst
RAG improved fact-based accuracy by fetching external knowledge, but it lacked reasoning capabilities. RAR fixes this by analyzing the retrieved information like a human expert would, leading to more meaningful insights, deeper understanding of complex questions, and reduction in irrelevant or misleading outputs.
Reduces Hallucinations in AI Responses
Traditional AI models often generate incorrect answers when they don’t have enough training data. RAR ensures factual accuracy by fetching real-time data, validating retrieved content through logical cross-checking, and reducing misinformation to increase reliability.
Enables Domain-Specific AI with Expert-Level Insights
RAR is particularly powerful in technical fields where deep analysis is required. In healthcare, it can analyze patient reports alongside the latest medical research. In legal applications, it can cross-reference case laws and apply legal reasoning. In finance, it can fetch and interpret stock trends for predictive insights. In scientific research, it can synthesize findings across multiple studies to provide comprehensive conclusions.
Personalized and Context-Aware Responses
Unlike static AI models, RAR adapts dynamically to different users and domains. If an AI assistant is being used in a legal firm, it can retrieve and analyze laws specific to a jurisdiction. If used in a customer support system, it can fetch and reason through relevant policies to give accurate solutions.
Challenges and Limitations of RAR
Despite its advancements, RAR is not without challenges.
Retrieval quality is a concern because if the AI retrieves low-quality or biased data, the reasoning process will also be flawed. This requires robust filtering mechanisms to ensure accuracy. Computational overhead is another challenge, as RAR models require more processing power than traditional AI, retrieving, filtering, and reasoning through data dynamically. Optimizing performance while keeping response times fast is an ongoing issue. Additionally, when different sources provide contradictory information, the AI needs to evaluate credibility and determine which data is most reliable an area still being refined.
The Future of Retrieval-Augmented Reasoning
RAR is still in its early stages, but AI researchers and developers are working on several improvements. Multi-modal RAR is being explored to allow AI to retrieve not just text but also images, graphs, and audio for richer responses. Self-learning retrieval is another area of development, where AI will improve its own reasoning by learning which sources are most reliable over time. Context-aware personalization is also a priority, ensuring that AI tailors responses based on individual user preferences and past interactions.
As AI becomes more integrated into business, research, and personal applications, RAR will redefine how AI interacts with knowledge making it not just a tool for answers, but a system for intelligent reasoning.
Final Thoughts: The Shift from Knowledge Recall to Intelligent Decision-Making
Traditional AI systems were great at recalling information, but they struggled with reasoning through complex ideas. RAR bridges this gap by making AI both factually accurate and analytically intelligent.
With RAR, AI moves from a simple information provider to a truly intelligent assistant, capable of deep analysis, logical reasoning, and high-precision decision-making. The future of AI isn’t just about finding information it’s about understanding, reasoning, and making sense of it in a way that helps users take informed actions.