Table Of Contents
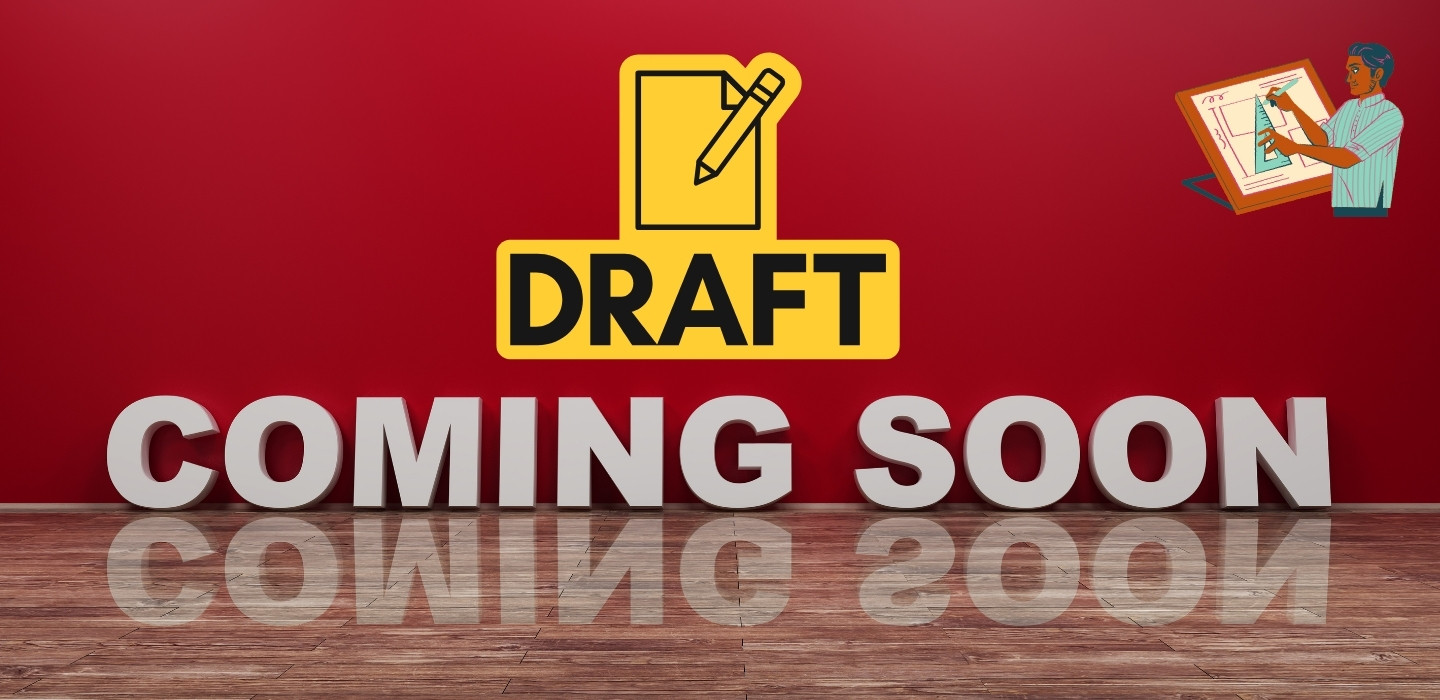
Artificial Intelligence is no longer a luxury; it is the backbone of modern applications. Whether you are building chatbots, recommendation engines, or fraud detection systems, AI-driven APIs make it possible to integrate powerful intelligence into your applications without building models from scratch. The key is knowing how they work, where to use them, and how to optimize them for performance and scalability. Let’s break it down in a way that is clear, practical, and professional.
What Makes AI-Driven APIs So Powerful?
Think of AI-driven APIs as a shortcut to intelligence. Instead of training a massive AI model, collecting data, and running computations, you send a request to an AI service it does the heavy lifting and returns the result.
These APIs are used across industries:
- E-commerce platforms use them for personalized recommendations.
- Banks and fintech companies rely on them for fraud detection.
- Healthcare applications use AI APIs to analyze medical images.
- Customer support systems deploy chatbots powered by NLP APIs.
Whether it is text, speech, images, or structured data, these APIs process real-world inputs and make applications smarter.
How AI-Driven APIs Work Behind the Scenes
To really use AI-driven APIs efficiently, you need to understand their workflow. It is not just about sending requests it is about optimizing responses, reducing latency, and ensuring cost-effectiveness.
Here is what happens when you interact with an AI API:
Input Data Is Sent
You send structured data (text, images, audio, video) via an HTTP request. This data could be:
- A text query for a chatbot API
- An image for an object detection API
- A voice clip for a speech-to-text API
AI Model Processes the Data
The AI model processes the input using deep learning models trained on vast datasets. Depending on the API, it might involve:
- Natural Language Processing (NLP) algorithms for text-related tasks
- Computer vision models for images and videos
- Neural networks for predictive analytics
The API Returns a Response
The API sends back a structured response usually in JSON or XML format. It might return:
- A translated text (in case of language APIs)
- A confidence score for a detected object (for image recognition)
- A probability of fraud (in financial applications)
Your Application Uses the Response
The final step is acting on the AI-generated insights. For example:
- A chatbot API generates a customer support response.
- A recommendation engine API suggests personalized products.
- A voice assistant API converts speech to actionable commands.
This cycle happens in milliseconds, making AI-powered applications fast, responsive, and intelligent.
Where Can You Use AI-Driven APIs?
AI-driven APIs are not just for big tech companies. Startups, enterprises, and even solo developers can integrate them into real-world projects.
Natural Language Processing (NLP) APIs
Used for: Chatbots, Sentiment Analysis, Text Summarization
Example APIs: OpenAI’s GPT API, Google Cloud NLP API
Best Practice: Fine-tune models for industry-specific applications. A generic chatbot might work well, but a customer service bot in finance or healthcare needs domain-specific training.
Computer Vision APIs
Used for: Image Recognition, Object Detection, Medical Imaging
Example APIs: Microsoft Azure Vision API, Amazon Rekognition
Best Practice: Reduce processing load by resizing and compressing images before sending them to the API. This speeds up responses and cuts costs.
Speech Recognition APIs
Used for: Voice Assistants, Call Center Automation, Subtitles
Example APIs: Google Speech-to-Text, IBM Watson Speech API
Best Practice: Ensure high-quality audio input. Background noise and poor microphone quality can reduce accuracy.
Recommendation System APIs
Used for: Personalized Content, Product Suggestions, Customer Retention
Example APIs: Amazon Personalize, Google Recommendations AI
Best Practice: Track user behavior over time to improve recommendation accuracy. Simple click-based recommendations do not work as well as behavioral-driven AI.
Predictive Analytics APIs
Used for: Fraud Detection, Demand Forecasting, Risk Assessment
Example APIs: Google AutoML, IBM Watson Predictive Analytics
Best Practice: Use custom datasets. Pre-trained models are great, but industry-specific data makes predictions far more relevant.
How to Implement AI-Driven APIs Like a Pro
If you are serious about building AI-powered applications, there are right and wrong ways to integrate APIs. Here is what expert engineers focus on:
Optimize API Calls to Reduce Costs
Most AI APIs charge per request. Calling them unnecessarily increases costs and slows down your application.
- Batch API requests instead of sending individual ones.
- Cache frequent responses so you do not make redundant calls.
- Use event-driven architectures to call APIs only when necessary.
Handle Errors Like a Pro
Not every request will be successful. APIs might return errors due to:
- Network failures
- Rate limits being exceeded
- Invalid input data
Make sure you log errors, retry failed requests, and implement fallback mechanisms so that your application does not break.
Secure Your API Access
Since AI APIs often process sensitive data, security should be a top priority.
- Use OAuth 2.0 or JWT authentication instead of exposing API keys in frontend code.
- Encrypt data before sending it to the API.
- Limit who has access to the API to prevent misuse.
Monitor API Performance Continuously
Performance bottlenecks can cause slow response times and higher costs.
- Use API analytics tools to track latency, failure rates, and API usage patterns.
- Scale API usage based on demand spikes to avoid unnecessary costs.
- Continuously test API responses for accuracy especially for evolving AI models.
Challenges to Watch Out For
AI APIs are powerful, but they come with challenges. Knowing these upfront helps you build more reliable applications.
Handling Latency Issues
AI processing takes time. Some APIs are faster than others, but real-time AI tasks like speech recognition or fraud detection require ultra-low latency.
- Use edge computing to process AI requests closer to users.
- Optimize API calls with asynchronous processing.
Avoiding Bias in AI Models
AI APIs are trained on massive datasets but that does not mean they are always unbiased.
- Regularly audit model outputs for biased predictions.
- Fine-tune models to align with fair and ethical AI principles.
Managing API Costs Effectively
Some AI APIs charge based on:
- Number of requests
- Complexity of computation
- Storage and processing time
To avoid surprises in billing:
- Set rate limits on API usage.
- Optimize how frequently you call AI APIs.
- Explore self-hosted AI models for cost-heavy tasks.
Final Thoughts
AI-driven APIs are changing how we build intelligent applications. They provide powerful AI capabilities without requiring deep expertise in machine learning. But to truly harness their power, you need to implement them efficiently, optimize for performance, and secure them properly.
By following best practices for API integration, cost management, and scalability, you can create AI-powered applications that are fast, intelligent, and future-proof. Whether you are building a chatbot, analyzing customer sentiment, or detecting fraud, AI-driven APIs are the key to next-generation automation and intelligence.