Table Of Contents
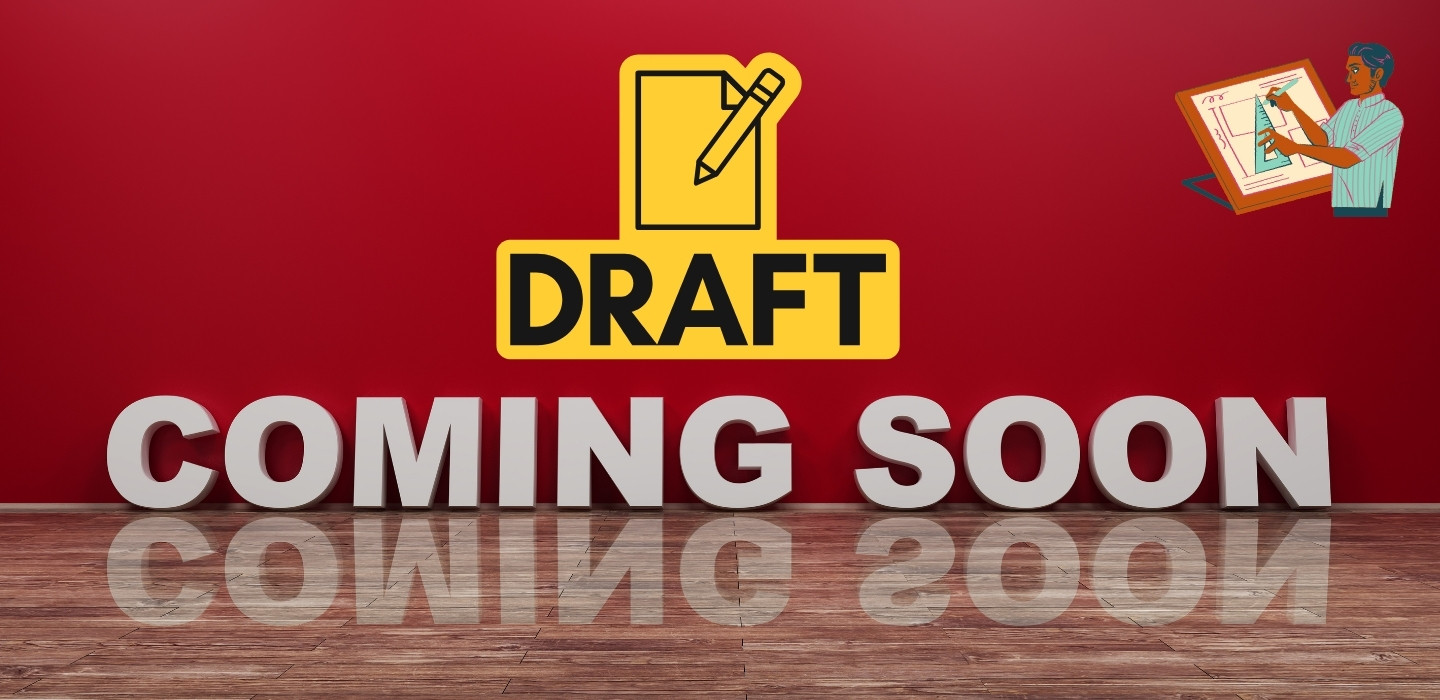
Artificial intelligence has advanced rapidly, but few innovations have had as much impact as the Transformer architecture. Introduced in 2017 by Google researchers in the paper “Attention is All You Need,” this model changed how machines process and generate language. Before Transformers, AI models relied on Recurrent Neural Networks (RNNs) and Long Short-Term Memory (LSTM) networks, which struggled with long sequences, required sequential processing, and took too much time to train. Transformers solved these problems by introducing self-attention and parallel processing, making AI models faster, more efficient, and better at understanding context.
Why the Transformer Architecture is Different
Traditional models like RNNs and LSTMs process data sequentially, meaning they handle one word at a time in order. While this works for short sequences, it becomes inefficient for longer texts because the model forgets earlier words or struggles to track complex relationships. Transformers changed this by processing entire sequences at once using self-attention. Instead of reading text step by step, the Transformer looks at all words at the same time and determines their relationships. This makes AI models much faster, more accurate, and capable of handling large amounts of text efficiently.
How the Transformer Model Works
The Transformer is built on two main components: the encoder and the decoder. The encoder processes input data and converts it into meaningful representations. The decoder takes these representations and generates a relevant output. This structure makes Transformers highly effective for tasks like language translation, text generation, and content understanding.
Key Components of the Transformer Model
Self-Attention Mechanism
The biggest innovation in Transformers is self-attention, which allows the model to determine how different words relate to each other, no matter where they appear in a sentence. For example, in the sentence “The dog chased the cat, and it ran away,” self-attention helps the model understand that “it” refers to “the cat” and not “the dog.” This is something older models struggled with because they processed words in a strict sequence.
Multi-Head Attention
Self-attention alone is powerful, but multi-head attention makes it even better. Instead of focusing on just one relationship at a time, multi-head attention allows the Transformer to look at multiple aspects of a sentence simultaneously. This helps the model better understand context and produce more accurate responses.
Positional Encoding
Since Transformers process all words at once, they need a way to understand word order. Positional encoding adds numerical values to each word so the model can keep track of sentence structure. This prevents confusion when processing phrases with different meanings depending on word order, such as “She only likes coffee” vs. “Only she likes coffee.”
Feedforward Layers
After the self-attention mechanism identifies relationships between words, the data is passed through feedforward layers to refine the output. These layers help the model understand patterns more deeply and ensure that responses are structured correctly.
Layer Normalization
Training AI models requires balancing information flow to prevent errors. Layer normalization ensures that all inputs remain stable, making training more efficient and improving model accuracy.
Why Transformers Are a Game-Changer for AI
Faster and More Efficient Processing
Unlike RNNs, which process text word by word, Transformers analyze entire sentences at once. This parallel processing makes them significantly faster, which is crucial for large-scale AI applications.
Improved Context Understanding
Because Transformers weigh relationships between all words in a sequence, they handle complex language structures better. This is why models like GPT-4, Google PaLM-2, and Meta’s LLaMA produce human-like text responses.
Scaling Up AI Models
Before Transformers, training AI on large datasets was too slow and inefficient. With the Transformer architecture, researchers can train models with billions or even trillions of parameters, leading to more powerful AI like ChatGPT, Bard, and Claude.
Real-World Applications of Transformers
Natural Language Processing (NLP)
Transformers power the most advanced NLP models today, enabling applications like chatbots, virtual assistants, and AI-driven content creation. They have dramatically improved machine translation, sentiment analysis, and automated summarization.
AI-Assisted Programming
Tools like GitHub Copilot and DeepMind AlphaCode use Transformers to help developers write, debug, and optimize code more efficiently. The AI understands programming logic and can generate code snippets based on user input.
Medical and Scientific Research
AI models trained with Transformer architecture assist in medical diagnostics, drug discovery, and genetic research. They can analyze massive datasets quickly, identify patterns, and even generate hypotheses for researchers.
Computer Vision and Multimodal AI
While Transformers started in language processing, they are now being used for image and video analysis. Models like DALL·E and Stable Diffusion generate images from text, proving that Transformers extend beyond just text-based AI.
Challenges and Limitations of Transformers
Despite their advantages, Transformers have some challenges:
High Computational Cost
Training large Transformers requires massive amounts of computing power, making them expensive to develop and deploy. Companies must optimize AI infrastructure to reduce costs.
AI Bias and Ethical Concerns
Since AI learns from human-generated text, it can inherit biases from its training data. Researchers must constantly refine models to ensure fairness and reduce harmful outputs.
Complexity in Fine-Tuning
Adapting Transformers for specific tasks requires extensive data and computational resources. Fine-tuning these models for different applications is still a challenge.
The Future of Transformer-Based AI
AI is evolving rapidly, and Transformers will continue to shape its future. Some key advancements on the horizon include:
- More Efficient AI Models – Future versions will require less computing power while maintaining high performance.
- Multimodal AI Integration – Transformers will become better at processing text, images, audio, and video together, improving AI’s real-world applications.
- Self-Learning AI – AI models will move toward continuous learning, where they improve dynamically based on real-time user interactions.
Final Thoughts: Why Transformers Matter
The Transformer architecture completely changed the AI landscape, making models faster, more scalable, and better at understanding human language. Without Transformers, breakthroughs like GPT-4, Bard, and LLaMA would not exist. These models power everything from AI chatbots and search engines to advanced research tools and creative content generation. As AI continues to evolve, Transformers will remain at the core of innovation, shaping the future of how humans and machines interact.