Table Of Contents
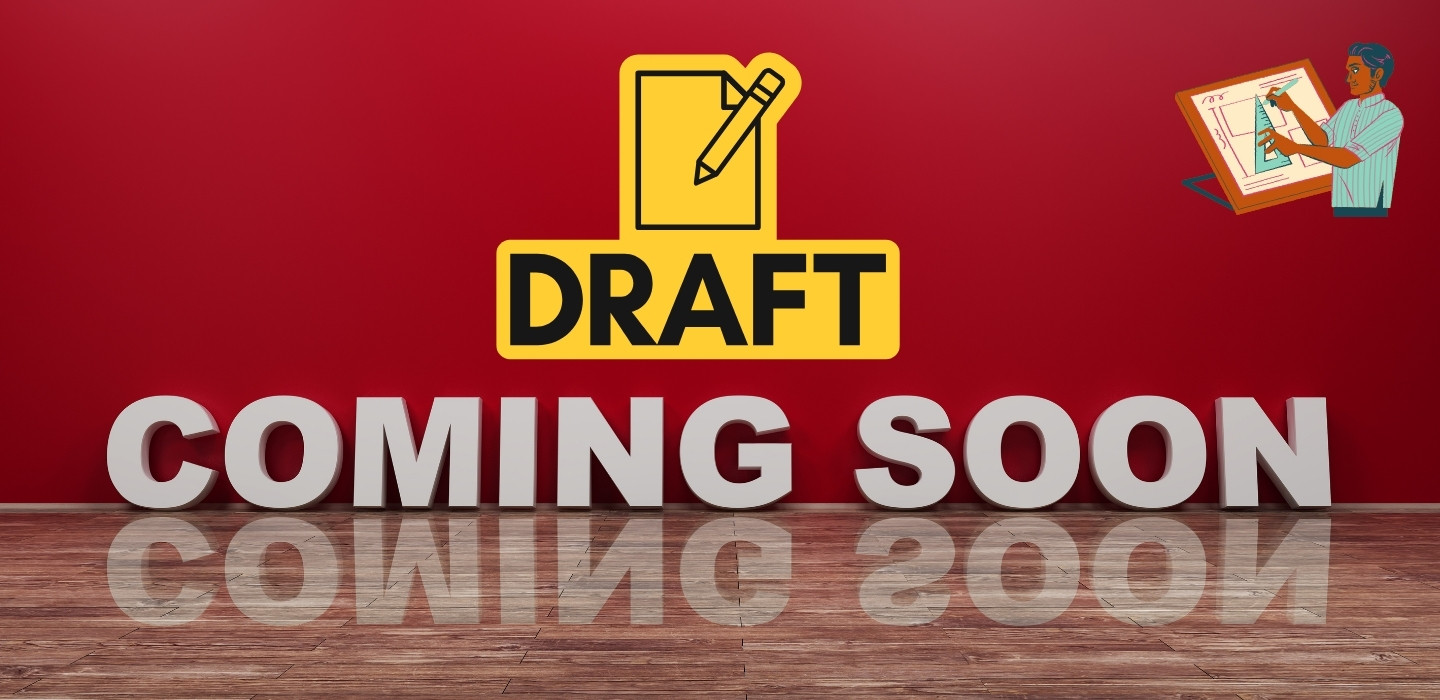
Introduction: The AI Revolution
Artificial intelligence has evolved rapidly, and one major breakthrough that changed everything is the Transformer architecture. Introduced by Google researchers in 2017, Transformers have reshaped how AI understands and generates language, images, and even complex reasoning tasks.
Before Transformers, AI struggled with understanding long pieces of text, slow training times, and difficulties processing large amounts of data. Older models like Recurrent Neural Networks (RNNs) and Long Short-Term Memory (LSTM) networks could only process information step by step, making them slow and inefficient.
The Transformer introduced self-attention mechanisms, allowing AI to process entire sequences at once. This breakthrough led to state-of-the-art language models like GPT-4, Google’s PaLM-2, and Meta’s Llama series, making it one of the most important advances in deep learning.
What is the Transformer Architecture?
A Transformer is a type of neural network designed to process and generate sequences of data, such as text or speech. Unlike older models that process information in order, Transformers analyze entire sequences at once, making them faster, more efficient, and better at understanding context.
Key Features of Transformers:
- Self-Attention Mechanism – Helps the model determine which words in a sentence are most important.
- Positional Encoding – Since Transformers process sequences all at once, they use positional encoding to understand word order.
- Multi-Head Attention – Allows the model to focus on multiple words at the same time, improving accuracy.
- Feedforward Layers – Additional processing layers that refine the model’s understanding.
- Layer Normalization – Helps the model train faster and work more efficiently.
By combining these features, Transformers can generate human like responses, translate languages, and even create images.
How Transformers Work
Transformers follow a structured process to understand and generate text, images, or other types of data. The model has two main parts:
- The Encoder – Reads and processes the input.
- The Decoder – Generates a response based on the processed information.
Step-by-Step Process:
Step 1: Tokenization & Embedding
The input (like a sentence) is broken into smaller parts called tokens. Each token is converted into numbers so the AI can process it.
Step 2: Positional Encoding
Since Transformers process entire sentences at once, they need to understand word order. Positional encoding ensures that “The cat sat on the mat” doesn’t get mixed up as “Mat the sat on cat the”.
Step 3: Self-Attention Mechanism
The model checks which words are important to each other. For example, in “The dog chased the ball, and it rolled away,” the AI needs to understand that “it” refers to the ball, not the dog.
Step 4: Multi-Head Attention
Instead of focusing on just one word at a time, the Transformer looks at multiple words at once, improving how well it understands meaning and context.
Step 5: Passing Through Feedforward Layers
After analyzing the input, the model refines its understanding by passing the information through deeper layers of processing.
Step 6: Output Generation
If the Transformer is translating a sentence or generating text, the decoder takes the processed information and creates a response that makes sense.
This structured approach allows Transformers to process large amounts of information quickly while keeping context and accuracy intact.
Why Transformers Changed AI Forever
1. They Solve the Problems of Older AI Models
Before Transformers, AI models had difficulty remembering long-term relationships between words and struggled with slow processing speeds. RNNs and LSTMs processed text word by word, making them inefficient for handling long passages.
Transformers solved these issues with self-attention and parallel processing, allowing them to handle entire paragraphs at once while maintaining accuracy.
2. They Power Today’s Most Advanced AI Models
Without Transformers, large AI models like GPT-4, Google’s PaLM-2, and Meta’s Llama wouldn’t exist. These models can:
- Generate human like text with incredible accuracy.
- Translate languages fluently while understanding context.
- Write code, answer complex questions, and assist in research.
3. They Train Faster and Process Data More Efficiently
Since Transformers process data in parallel, they train much faster than older models. This allows AI companies to develop powerful models in weeks instead of years.
For example, GPT-3 has 175 billion parameters, which would have been impossible to train using older architectures like RNNs.
4. They Work With More Than Just Text
Transformers aren’t limited to just language. They also power AI models that process images, videos, and speech. Some real-world examples include:
- DALL·E – Generates images from text descriptions.
- Whisper – Converts spoken words into text.
- Stable Diffusion – Creates high-quality digital art based on prompts.
This flexibility makes Transformers the foundation of modern AI research and development.
Challenges of Transformers
Even though Transformers are powerful, they also have some limitations:
1. They Require Massive Computing Power
Training large Transformers requires huge amounts of computing power and memory, making them expensive to run.
2. They Use a Lot of Energy
Since they rely on billions (or even trillions) of parameters, Transformers consume large amounts of electricity, raising concerns about their environmental impact.
3. They Can Inherit Biases
Because Transformers learn from large datasets on the internet, they can pick up biases present in that data. Developers must fine-tune and monitor models to ensure fairness and avoid harmful outputs.
4. They Are Expensive to Train and Maintain
Fine-tuning a Transformer for specific tasks requires enormous amounts of labeled data, which can be costly and time-consuming.
Despite these challenges, researchers are working on making Transformers more efficient with methods like sparse attention and retrieval-augmented models.
The Future of Transformers in AI
Transformers will continue to drive the future of AI with exciting advancements, including:
- Smaller, More Efficient Models – AI that runs on smartphones and edge devices without needing massive data centers.
- Multimodal AI – AI that can seamlessly understand text, images, and audio at the same time.
- Self-Improving AI – Future Transformers that learn from real-world interactions and get better over time.
As AI becomes more powerful, Transformers will be at the heart of everything from smarter chatbots to medical breakthroughs.
Final Thoughts: Why Transformers Matter
Transformers are the foundation of today’s AI breakthroughs, enabling everything from chatbots and search engines to image generation and speech recognition. By solving the limitations of older AI models, they have made AI faster, more scalable, and capable of truly understanding human language.
As AI research continues, Transformers will shape the next generation of artificial intelligence, driving innovation in medicine, education, business, and entertainment.