Table Of Contents
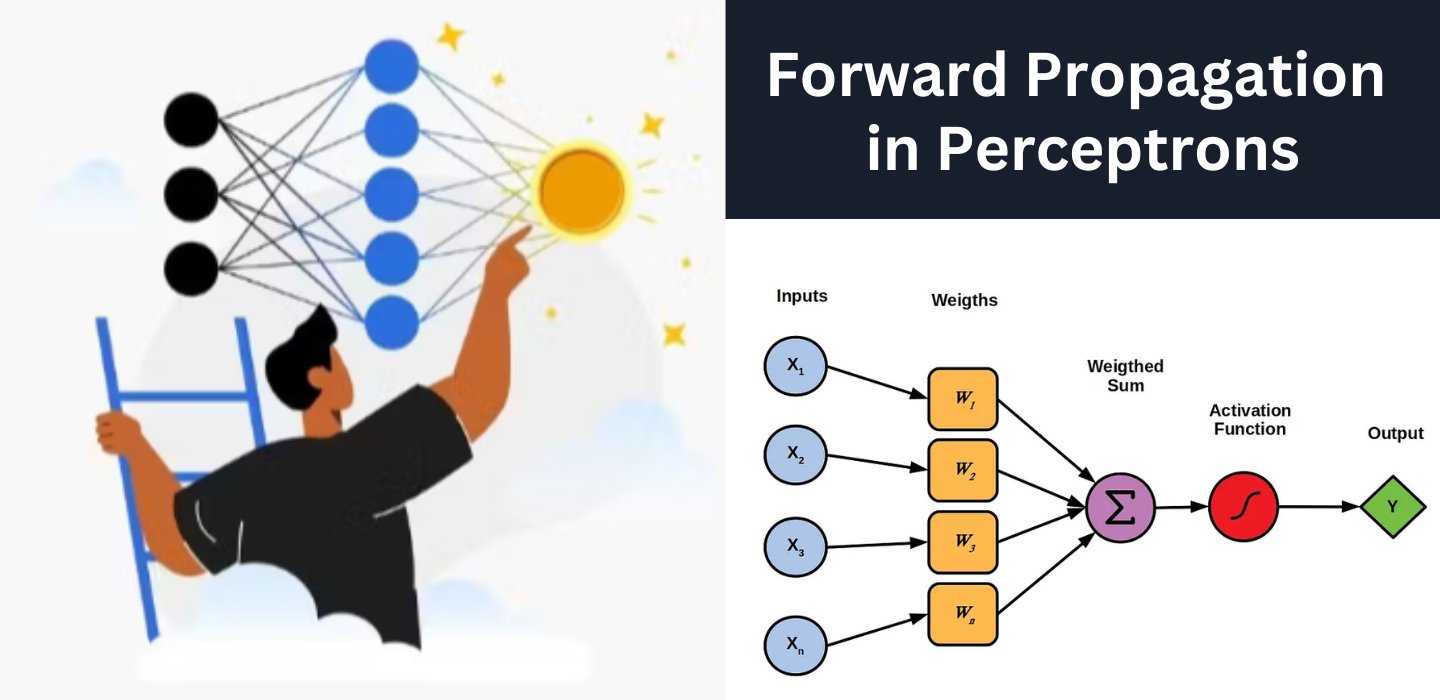
The Perceptron: Understanding Forward Propagation in Neural Networks
The perceptron is the foundation of artificial neural networks. It is the simplest type of artificial neuron and serves as the building block for more complex deep learning models. Understanding forward propagation in a perceptron helps in grasping how neural networks process information, learn patterns, and make predictions.
What is a Perceptron?
A perceptron is a computational model inspired by biological neurons. It takes multiple inputs, applies weights, sums them, and passes the result through an activation function to determine an output. The perceptron was first introduced by Frank Rosenblatt in 1958 as an early attempt at machine learning, capable of solving simple classification tasks.
How Forward Propagation Works in a Perceptron
Forward propagation is the process of passing input data through the perceptron to compute an output. This is the first step in training a neural network before backpropagation is applied to adjust weights. The process involves three main steps:
1. Weighted Sum Calculation
Each input xix_ixi is multiplied by a corresponding weight wiw_iwi. The perceptron then sums these weighted inputs along with a bias term. Mathematically, this is represented as:
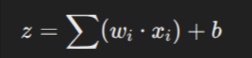
Where:
- xix_ixi are the input values
- wiw_iwi are the associated weights
- bbb is the bias term (helps shift the activation function)
- zzz is the weighted sum
The weights determine the importance of each input. Higher weights indicate stronger influence on the perceptron’s decision.
2. Activation Function Application
Once the weighted sum is calculated, it is passed through an activation function. The activation function introduces non-linearity, allowing the perceptron to learn complex patterns.
For a basic perceptron, the most common activation function is the step function, which outputs either 0 or 1 based on a threshold:
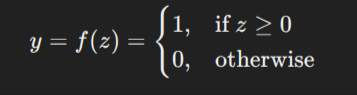
However, modern neural networks use other activation functions like sigmoid, ReLU, and tanh for better learning capabilities.
3. Output Decision
The final step is determining the output. If the activation function’s result meets a certain threshold, the perceptron outputs 1 (positive class); otherwise, it outputs 0 (negative class). This binary classification approach is the basis of early machine learning models.
Understanding the Role of Forward Propagation
Forward propagation in a perceptron is deterministic, it follows a fixed set of operations from input to output. It does not learn during this phase; it only processes data based on current weights. The learning happens when backpropagation adjusts the weights to minimize errors.
Limitations of a Single-Layer Perceptron
While the perceptron can classify linearly separable data, it fails on non-linearly separable problems like XOR. This limitation led to the development of multi-layer perceptrons (MLPs) and deep learning architectures, which use multiple layers and activation functions to solve complex problems.
Why Forward Propagation is Essential in Deep Learning
Understanding forward propagation in a simple perceptron builds the foundation for deep learning models. Every neural network, no matter how complex, follows the same principles, taking inputs, applying weights, passing them through activation functions, and producing outputs. In deep networks, forward propagation happens across multiple layers, with each layer extracting deeper features from the data.
Final Thoughts
The perceptron introduced the idea of machine learning through mathematical computations and decision boundaries. Forward propagation remains the backbone of all neural networks, enabling AI models to process information and make predictions. While simple perceptrons are rarely used in real-world applications today, their principles still power modern deep learning.
Understanding these basics is crucial for anyone working in AI, as it forms the core of how models recognize patterns, classify data, and evolve through learning algorithms.